What Type of Data Includes Text Messages, Social Media Data, and Email? Explained Simply
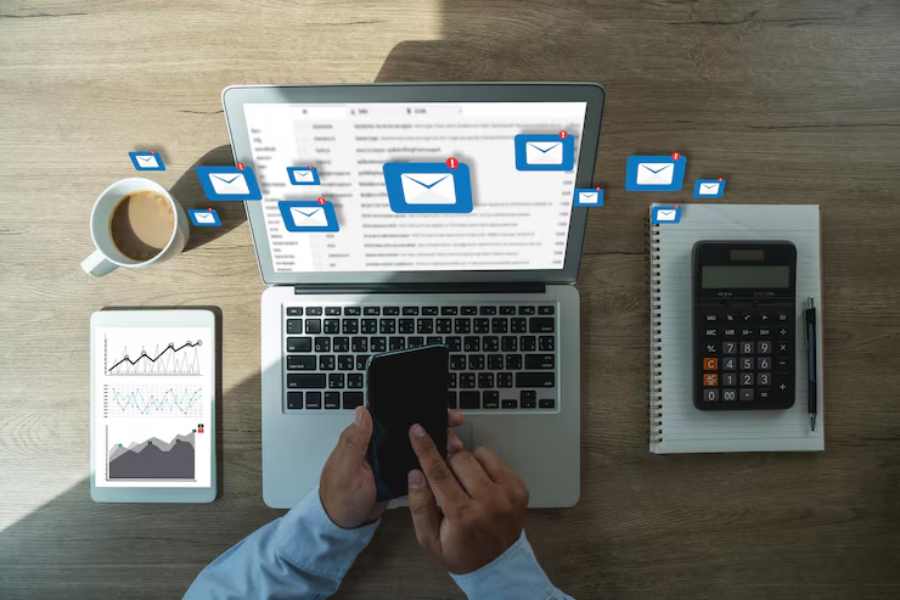
In the digital age, data flows in countless formats—from structured rows in databases to spontaneous, user-generated content like texts, emails, and social media posts. But what type of data includes text messages, social media data, and email? This question isn’t just technical—it’s vital for businesses, legal professionals, marketers, and cybersecurity teams.
Understanding what category these types of content fall under helps with proper storage, legal compliance, and strategic analysis. All three—text messages, social media content, and email—belong to a broader classification called unstructured data. This data type doesn’t follow a conventional format and can’t be easily entered into a relational database. Yet, it’s packed with valuable information, from customer sentiment to business insights and even legal evidence.
From archived tweets to email chains and text logs, unstructured data now makes up more than 80% of all enterprise data. However, its fluid and often informal nature makes it difficult to manage. Unlike structured data, it requires advanced tools and strategies to extract meaning.
This article will break down what type of data includes text messages, social media data, and email, why it matters, and how organizations should handle it. We’ll explore data types, classification challenges, real-world applications, and best practices to secure and analyze these digital communication formats effectively.
What type of data includes text messages, social media data, and email?
These types of content are classified as unstructured data. Unlike structured data in databases, unstructured data includes free-form text, images, and communication formats like emails, texts, and social posts. It’s rich in information but requires specialised tools to process and analyse effectively.
Why Understanding Unstructured Data Matters in the Digital World
In today’s digital-first world, understanding what type of data includes text messages, social media data, and email is crucial for both individuals and organisations. These communication formats are everywhere—powering personal chats, customer service, marketing campaigns, and legal records. However, they differ significantly from traditional, structured data found in spreadsheets and databases.
Text messages, social media interactions, and emails fall into the category of unstructured data. This type of data is not organised in a predefined format, making it more complex to store, retrieve, or analyse. A tweet, for example, may contain text, hashtags, emojis, and links—all varying in context and emotional tone. It’s this richness of detail that gives unstructured data its value but also its challenges. Sometimes, content may even be disguised or obscured visually, similar to the effects seen when using an invisible text generator, where the presence of data isn’t immediately obvious but still exists within the digital structure.
Businesses use unstructured communication data to monitor customer sentiment, detect brand trends, and craft targeted marketing strategies. Legal teams depend on it for evidence in litigation. Cybersecurity teams analyze it for policy violations or potential threats. Yet, many organisations fail to manage it properly due to its sheer volume and irregular structure.
Failing to grasp what type of data includes text messages, social media data, and email can lead to missed insights, non-compliance risks, and poor decision-making. In a world fueled by conversations and digital expression, understanding and managing unstructured data has become a modern necessity.
How to Classify Data Types for Better Management
Understanding how to classify data types is essential for effective digital organisation and management. In today’s world, where data is constantly being generated across platforms and devices, distinguishing between different types of data allows for better storage, analysis, and decision-making.
Structured vs. Unstructured Data
Structured data is the most straightforward to manage. It resides in clearly defined fields within relational databases, such as names, dates, and numbers in spreadsheets or SQL databases. This kind of data is highly organised, making it easy to search, sort, and analyse using traditional data tools.
On the other hand, unstructured data lacks a predetermined format. Examples include emails, text messages, videos, audio files, and social media posts. These types of data do not fit neatly into tables or rows, which makes them harder to process with conventional tools. Despite their complexity, unstructured data often holds valuable insights because of its richness and context.
What Is Semi-Structured Data?
Semi-structured data sits between structured and unstructured data. It does not follow a rigid structure like a database, but still includes tags or markers to separate elements and enforce hierarchies. Formats such as XML, JSON, and Nosql databases fall into this category. These allow for a degree of organisation without being confined to a strict schema, providing more flexibility.
The Importance of Metadata
Metadata plays a critical role in managing unstructured and semi-structured data. Information like timestamps, author names, file sizes, and geolocation details adds a layer of structure that makes the content more searchable and sortable. Metadata acts as a bridge, enabling organisations to make sense of otherwise disorganised information.
Common Examples of Unstructured Communication Data
Unstructured communication data encompasses a wide variety of formats that don’t follow a consistent structure, yet they carry valuable context and insights. Below are some of the most common examples found in both personal and professional environments:
- Text Messages: These are short, real-time exchanges between users that often include informal language, abbreviations, emojis, and slang. Their spontaneity and lack of formatting make them a key example of unstructured communication.
- Social Media Posts: Content from platforms like Facebook, Instagram, Twitter (X), or TikTok includes text, hashtags, photos, videos, reactions, and links. Each post varies greatly in format, tone, and media, contributing to its unstructured nature.
- Emails: Emails combine body text, subject lines, headers, metadata, and attachments. While email clients impose some consistency, the open-ended structure of messages and attachments keeps them in the unstructured category.
- Voicemail Transcripts: Converting voice messages into text introduces variability in sentence structure and interpretation. These transcripts often contain errors or inconsistencies due to speech recognition limitations, making them unstructured by nature.
- Instant Messaging Apps: Applications like WhatsApp, Slack, Microsoft Teams, and Facebook Messenger feature text, voice notes, images, documents, reactions, and stickers. The flow of conversation is dynamic and not organized by formal rules.
- Customer Support Chats: These chats capture dialogues between customers and representatives. They often include misspellings, unclear phrasing, and emotional expressions, making them difficult to categorise but crucial for understanding customer behaviour.
Why Is Unstructured Data Harder to Analyse Than Structured Data?
Unlike structured data that fits neatly into tables, unstructured data lacks uniformity. Each email, text, or tweet can differ in length, tone, and format. Natural language adds layers of complexity—sarcasm, idioms, and multilingual content make automated interpretation difficult. Additionally, embedded elements like emojis, images, and links create parsing challenges.
Another hurdle is inconsistency in storage. Structured data lives in databases. Unstructured data may exist in email inboxes, chat logs, cloud platforms, or social media APIS—often unlinked or scattered. Without centralisation, analysis becomes time-consuming and incomplete.
Even when gathered, this data requires specialised tools like natural language processing (NLP) and machine learning to interpret patterns or sentiments. This is why many organisations turn to AI-powered solutions to extract usable insights from unstructured formats. Data privacy regulations like GDPR also add complexity, requiring companies to anonymise or secure communication data before processing.
Managing what type of data includes text messages, social media data, and email requires a strategic mix of technology, compliance, and contextual understanding.
Best Practices for Handling Communication-Based Unstructured Data
Managing unstructured communication data—like emails, texts, chats, and social media interactions—requires a thoughtful strategy that balances organisation, privacy, and technological support. Here are the best practices to effectively handle this type of data:
- Create a Centralised Storage System: Start by consolidating all communication data into a single cloud repository or platform. Centralisation ensures faster access, simplifies searchability, and enables more consistent data management across teams and departments.
- Use Metadata to Your Advantage: Metadata such as timestamps, sender information, locations, and device types can bring structure to otherwise disorganised content. By tagging and organising data with metadata, businesses can make unstructured data easier to categorise, retrieve, and analyse.
- Apply AI and NLP Tools: Leverage artificial intelligence and natural language processing (NLP) to extract meaning from large volumes of text. These tools can identify recurring themes, extract keywords, recognise sentiment, and even detect trends, making the data more actionable.
- Monitor Privacy Compliance: Always stay aligned with global data protection laws like GDPR and CCPA. Communication data should be encrypted, anonymised where appropriate, and collected or used only with proper consent. Failing to comply can result in serious legal and financial consequences.
- Train Teams on Data Sensitivity: Ensure that employees handling communication data understand its importance and sensitivity. Training should cover legal risks, ethical use, and the business value of properly managing unstructured data.
Final Thoughts
Recognising what types of data include text messages, social media data, and email is essential in today’s data-driven world. These formats are classified as unstructured data, rich in value but complex to manage. While structured data fits neatly into databases, unstructured communication requires advanced tools like AI and NLP to unlock insights. From customer sentiment to legal compliance, these messages hold strategic importance across industries. However, managing them demands secure storage, metadata tagging, and a focus on privacy regulations. By understanding and organising this data effectively, businesses can improve decision-making, strengthen customer relationships, and stay compliant. In short, unstructured data isn’t a burden—it’s a powerful asset when handled with the right approach.
FAQ’s
Q. What type of data includes text messages, emails, and social posts?
A. These fall under unstructured data, which doesn’t follow a predefined format and includes various communication forms.
Q. Is unstructured data useful in business intelligence?
A. Yes, analysing unstructured communication like customer emails or social comments reveals trends, feedback, and user sentiment.
Q. What tools can analyse unstructured data?
A. Natural language processing (NLP), AI, and machine learning platforms like IBM Watson or Google Cloud AI can process unstructured text.
Q. Why is structured data easier to manage than unstructured data?
A. Structured data is uniform and stored in databases, making it easier to search, query, and organise compared to unpredictable text formats.
Q. Can unstructured data be secured and stored legally?
A. Yes, but it must comply with privacy laws. Use encryption, access control, and data masking to protect sensitive communication content.
Q. Are images and videos considered unstructured data, too?
A. Yes. Visual content like memes, screenshots, and videos from social platforms also falls under unstructured data.